Research
Metabolomics Data Harmonization and Meta-Analysis

Liquid chromatography tandem mass spectrometry (LC-MS) has emerged as the major technology used for metabolomic profiling, however raw datasets require extensive processing before they may be analyzed toward discovering biological patterns and disease associations.
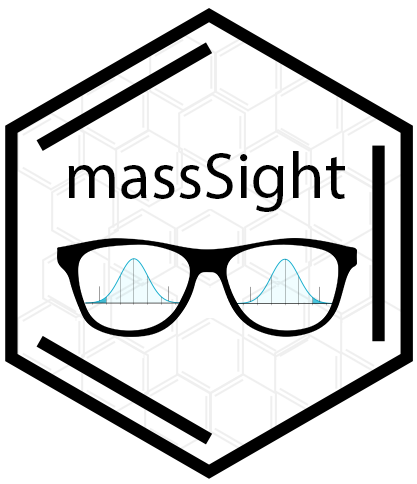
We are currently developing massSight, an R package for the alignment and scaling of LC-MS data. We seek to produce a suite of statistical and computational methods to increase the biological signal-noise ratio. These approaches include removing batch effects, drift correction, clustering chemical compounds, and removal of non-biological background signals.
AI/ML to Infer Biology from Sequencing Data
The application of Artificial Intelligence (AI) and Machine Learning (ML) in deciphering biological insights from sequencing data represents a transformative shift in the field of Computational Biology. These computational technologies can handle the enormous volumes of data generated by sequencing platforms, from DNA and RNA to more complex proteomic sequences. Traditional analytical methods often fall short of capturing the intricate patterns and relationships hidden in this data. AI/ML algorithms, however, excel in identifying these subtle connections, enabling more accurate predictions and fostering deeper understanding of biological processes. We are developing AI and ML models and tools for applications ranging from profiling microbial species and biosynthetic gene clusters (BGCs), identifying genetic markers for diseases, testing for rare diseases, understanding evolutionary pathways, to even the development of personalized medicine.
deepBreaks
seqLens
BGCLens
ResLens
Characterizing temporal dynamics of longitudinal omics
Longitudinal studies and clinical trials, combined with omics measurements, are revolutionizing drug development by providing a holistic understanding of disease progression, treatment responses, and shifts in biological markers. This integration accelerates drug discovery and enables the utilization of advanced technologies like AI and machine learning. However, challenges such as complex data structures and limited sample sizes can restrict the full potential of longitudinal omics data. To overcome these challenges, we aim to develop robust machine learning techniques, including Gaussian Processes, tailored for longitudinal omics analysis. Our strategy involves the creation of user-friendly software such as wavome and the application of these methodologies to enhance biomarker discovery and association testing by characterizing the dynamics of omics features in relation to clinical and participant/sample characteristics.
wavome
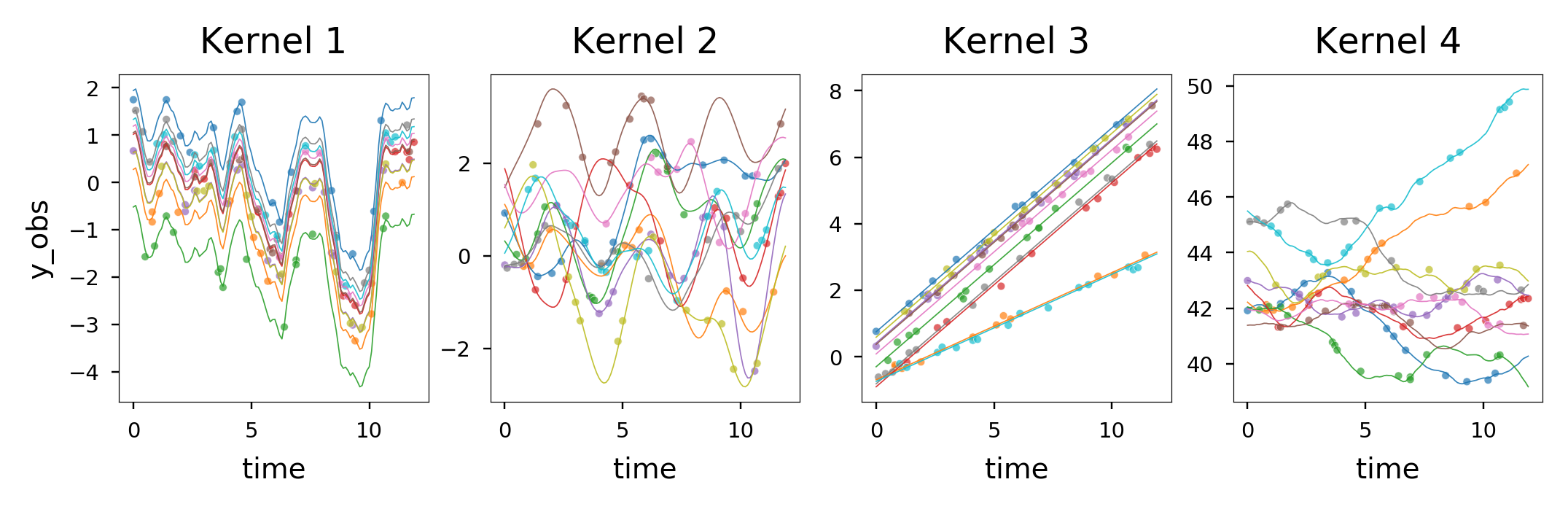
Functional Integration of Omics Data
Recent advancements in high-throughput technologies, such as DNA sequencing techniques and liquid chromatography-mass spectrometry, have enabled us to capture intricate snapshots of human biology activities through multi-omics data on a large scale. This multi-omics approach provides an unprecedented opportunity for in-depth structural and molecular profiling of human biology across various molecular levels. However, the challenge lies in effectively integrating and analyzing this wealth of multi-omics data.
These modern biological screens produce an overwhelming number of measurements, spanning genomics, transcriptomics, proteomics, and metabolomics, among others. Finding statistically significant associations among features and integrating these different omics data sets at the metabolic functional level in an interpretable manner is imperative.
In this project, our goal is to develop statistical and machine learning tools that leverage deep learning approaches to harness the power of multi-omics data. We aim to uncover enriched metabolic pathways and gain a comprehensive understanding of human biology by integrating information across these diverse molecular layers. This addresses the critical need for more efficient and insightful analysis of complex biomedical data, particularly in the context of multi-omics datasets.
btest omePath
Investigating human health conditions using omics data
COVID-19
The COVID-19 pandemic, driven by the SARS-CoV-2 virus, has brought about profound global changes, yet its ultimate consequences remain uncertain. As the virus evolves in response to host immune systems and intervention measures, efforts are underway to develop accessible, repeatable tools for integrating and analyzing the vast array of pandemic-related data. These tools are being applied to study genetic variations in SARS-CoV-2 and their associations with clinical health outcomes. Additionally, metabolomics and proteomics data are being employed to understand changes in COVID-19 severity. These endeavors aim to provide valuable insights for guiding vaccine development, monitoring disease epidemiology, and characterizing the virus’s genomic evolution patterns.
In parallel, research efforts are exploring the interplay between the viral genome and host genetic backgrounds, examining the 3D protein structure’s role in disease etiology, and investigating biomarkers that explain the diverse health outcomes associated with COVID-19. Deep sequencing analysis and machine learning techniques are being employed to identify regions in the gene sequence responsible for COVID-19 detection, while omics technologies are being leveraged to link genetic and phenotypic data with clinical information for disease prediction, diagnosis, and therapeutic advancements. These multifaceted research initiatives aim to provide valuable resources and insights to the broader scientific community, facilitating collaboration and discussions to combat the pandemic effectively.
Microbiome and Metabolomics of Pregnancy, Breast Milk Feeding, and Infant Health
The microbial ecosystem within human breast milk plays a crucial role in shaping an infant’s early gut microbiome, influencing their long-term health. Despite the growing interest in this intricate relationship, a significant knowledge gap exists regarding the dynamics between breast milk and the infant gut microbiome and metabolome. To address this deficit, we conducted a comprehensive study within the neonatal intensive care unit (NICU) and among participants from the breast milk B12 concentration of participants from clinical trials. We collected paired samples of breast milk and infant stool from infants during their first 15 weeks of life. Leveraging shotgun metagenomic sequencing and untargeted metabolomics, we delved into the intricate world of microbial and metabolic interactions. In our exploration, we uncovered intriguing associations between maternal clinical factors, such as BMI, and breast milk microbiome diversity. Surprisingly, our findings challenge the prevailing notion of extensive colonization of breast milk microbiota within the infant gut. This study not only characterizes the breast milk microbiome but also sheds light on the complex interplay between maternal factors and the infant’s early microbial landscape.
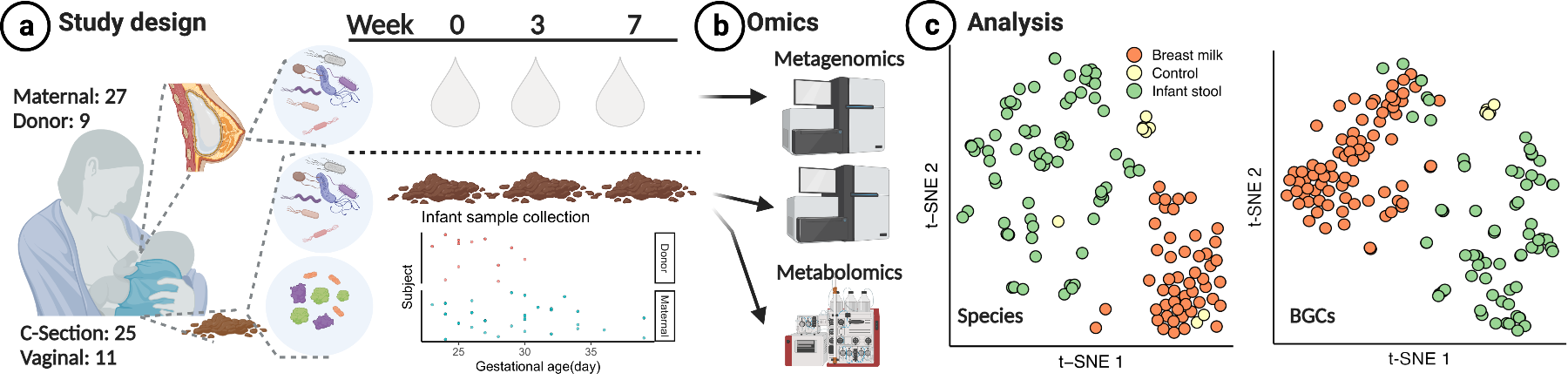
Microbiome and Metabolomics of Cancer
NF1 is a syndrome caused by NF1 gene inactivation, leading to increased cancer risk and metabolic issues due to overactive MAPK signaling. MEK-inhibitors (MEKi) were approved for NF1-related tumor treatment, but their impact on metabolism remained uncertain. Our study, using Nf1-/- mice and human patient data, reveals that MEKi treatment swiftly affects long-chain fatty acid metabolites. Long-term MEKi treatment may influence biomarkers tied to energy conversion and neurological health. Interestingly, most effects revert after treatment cessation. These findings suggest a potential link between MEKi use and reversing NF1-associated metabolic dysfunction, warranting further investigation.
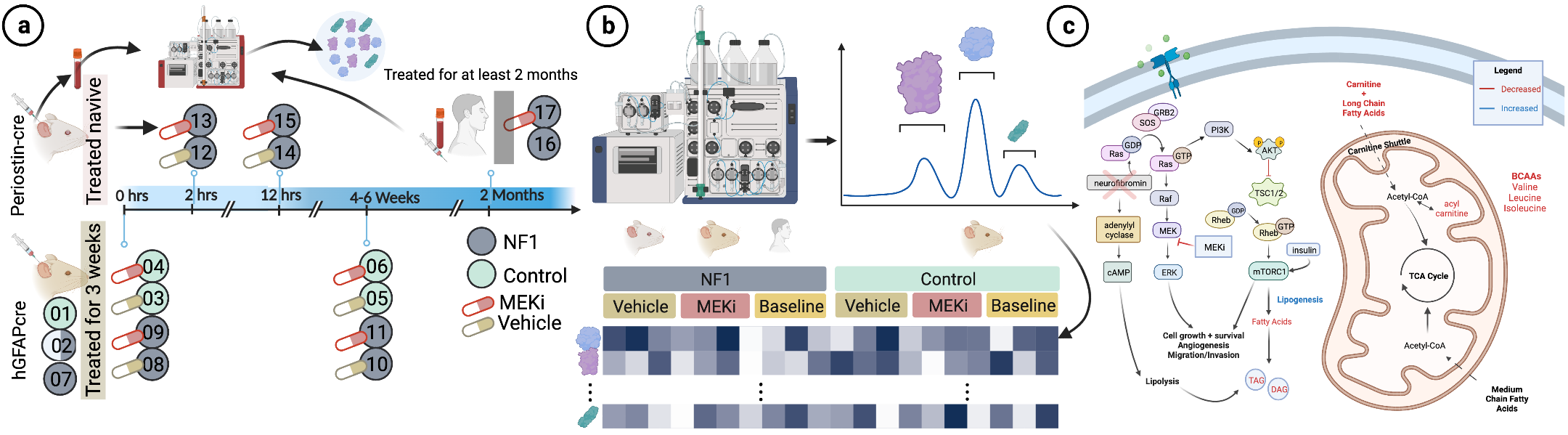
Microbiome and Metabolomics of Obesity, RYGB, and Sleeve
Exploring molecular pathways in Roux-en-Y gastric bypass (RYGB) for obesity and diabetes therapy, we performed integrated proteomics and metabolomics on obese rats undergoing RYGB, sham surgery, or calorie restriction. Key factors involved glucose and lipid metabolism proteins (e.g., Apolipoprotein E, Phosphoglucomutase−1), immunity elements (e.g., complement system), and extracellular matrix components (e.g., type I collagen), with sphingomyelin downregulation central to RYGB dysregulation. This study sheds light on post-RYGB circulating metabolite and protein changes, revealing functional dynamics. In parallel, we investigate alpha/beta diversity changes post-Sleeve gastrectomy (SG) in severely obese adolescents compared to controls at 1 and 2 years, examining microbiome alterations, correlations with insulin metrics, metabolite associations, and bone measurements, providing insights into SG’s impact over time.
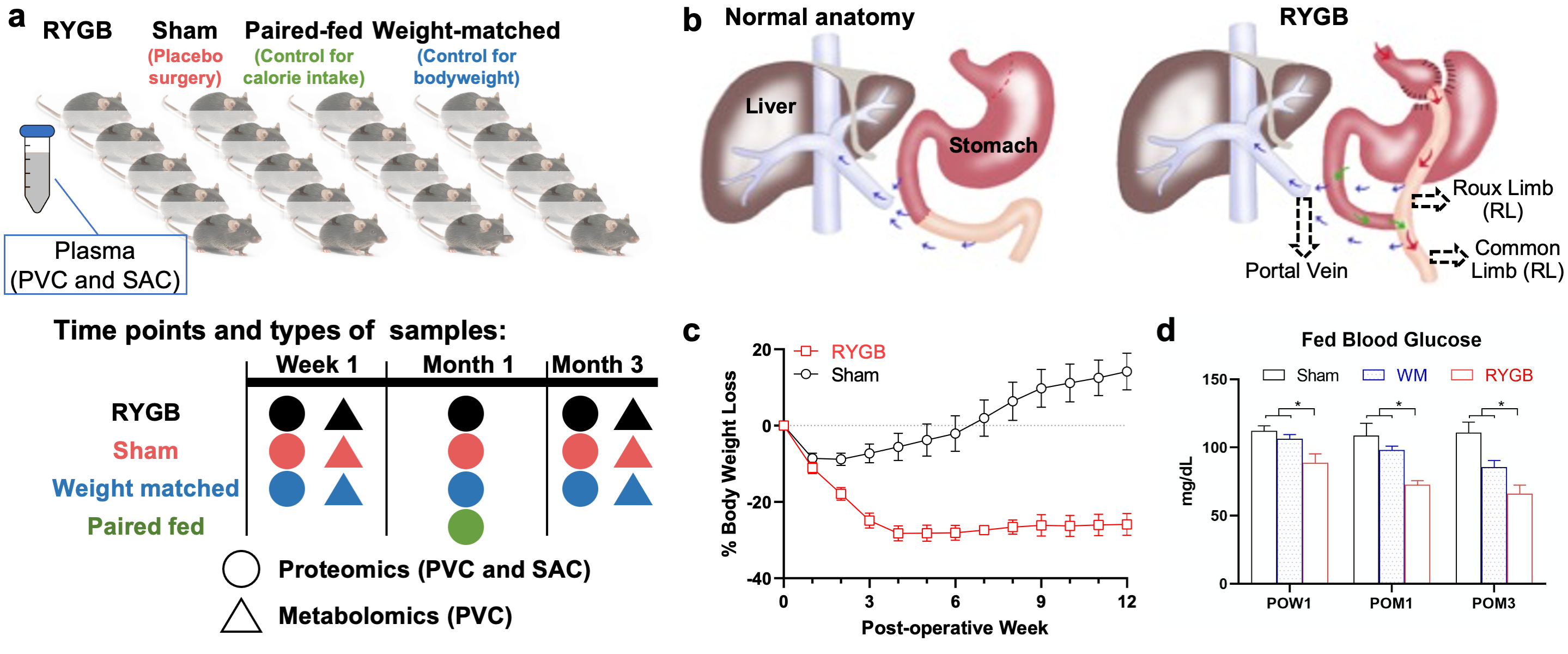
Characterizing Dynamics of Cells and Genes in Skin Injury
This study delves into the mechanisms of early skin wound healing, focusing on cellular communication networks during the initial inflammatory stages. Leveraging single-cell RNA sequencing, we examine changes in gene expression in injured skin compared to non-injured controls. Our pipeline, cellSight, employs advanced tools for clustering, integration, and differential gene expression analysis. We aim to identify precise cell populations, investigate cell-to-cell communication during early inflammation, and pinpoint key genes marking biological changes post-injury. Understanding these processes is critical for improving wound healing strategies and promoting tissue repair.
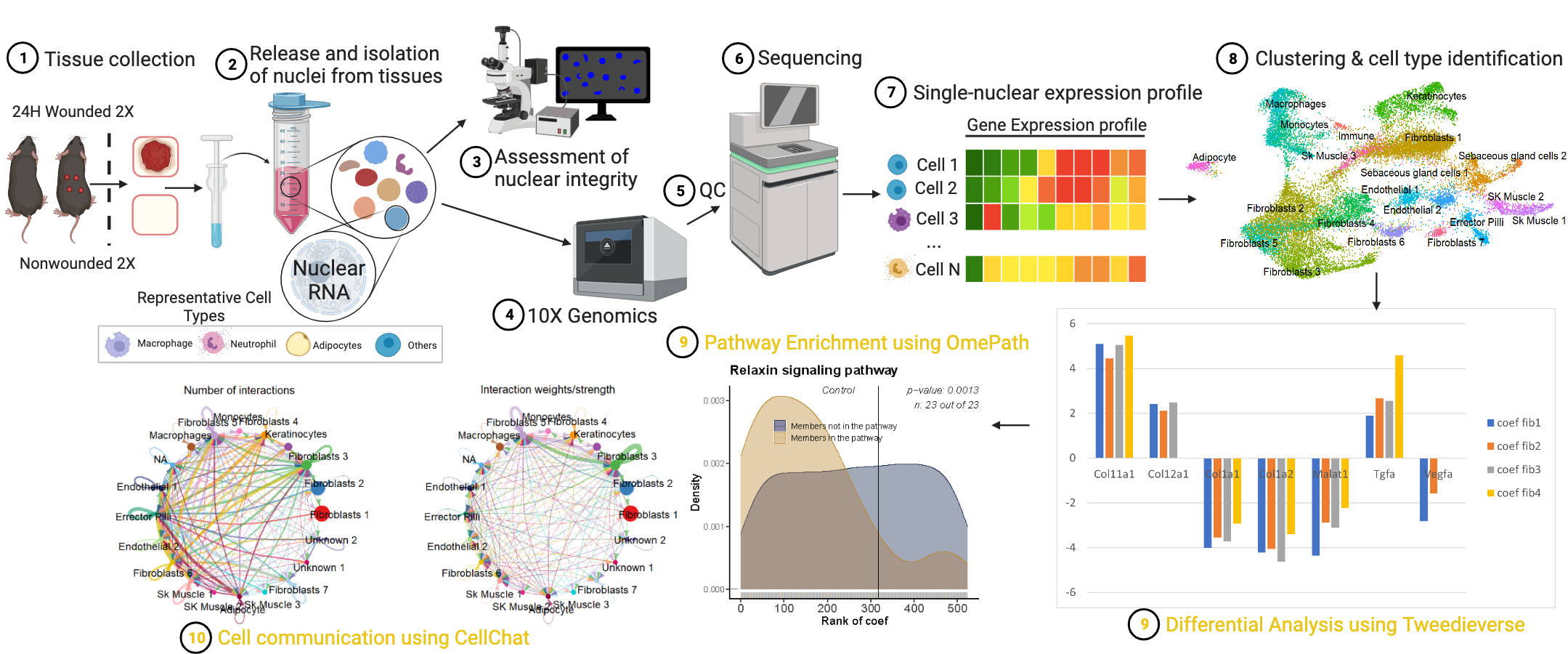